Modeling the Relationship between Process Parameters and Bulk Density of Barium Titanates
Article information
Abstract
The properties of powder metallurgy products are related to their densities. In the present work, we demonstrate a method to apply artificial neural networks (ANNs) trained on experimental data to predict the bulk density of barium titanates. The density is modeled as a function of pressure, press rate, heating rate, sintering temperature, and soaking time using the ANN method. The model predictions with the training and testing data result in a high coefficient of correlation (R2 = 0.95 and Pearson’s r = 0.97) and low average error. Moreover, a graphical user interface for the model is developed on the basis of the transformed weights of the optimally trained model. It facilitates the prediction of an infinite combination of process parameters with reasonable accuracy. Sensitivity analysis performed on the ANN model aids the identification of the impact of process parameters on the density of barium titanates.
1. Introduction
Barium titanate (BaTiO3) is one of the most widely used ceramics in the electronic industry due to their simple crystal structure and superior dielectric properties [1]. The high dielectric constant of barium titanate makes an attractive material to use as capacitors, medical imaging, ultrasonic cleaning, gas lighters, underwater detection, and other applications. The bulk density and porosity of barium titanate influence the dielectric properties, and high density and low porosity are favorable for high dielectric properties. Sintering BaTiO3 at high temperature with long soaking time to achieve high density is standard practice. The synthesis processes for BaTiO3 require expensive precursors or organic solvents, complicated steps, and long reaction times and very costly to conduct experiments and to achieve the results, hence developing the predictive models is much required. Recently, artificial neural networks (ANN) technologies are used in various fields of powder technologies [2-8]. ANN models create a correlation between input and target output with the help of hidden neurons in hidden layers [9]. The objective of the present study was to develop an ANN model to predict and analyze the bulk density of barium titanates as a function of pressing pressure, pressing rate, sintering temperature, sintering rate, and soaking time.
2. Materials and Methods
The experimental data of the present work are collected from the published literature [10]. BaTiO3 powder was prepared by a modified Pechini method as described elsewhere. Five different batches with different concentrations of barium chloride and titanium chloride were prepared to achieve different particle sizes [10]. The input parameters of the model were; the magnitude of pressing pressure, pressing rate, the temperature of sinter-ing, rate of sintering, and soaking times, and the one output parameter is bulk density. The number of samples that were used for training the model was 60, while 20 samples were employed for testing the performance of the trained model. The statistics of the data used for ANN model development was shown in Table 1.
In the present work, ANN trained with the backpropagation algorithm (BPA), and the information as vastly available and summarized elsewhere [11, 12]. The model was executed using the programming languages C and Java. Figure 1 shows the schematic diagram of the present problem. It consists of five nodes representing process parameters at the input layer and one node at the output layer. In between these two layers are the hidden layers with several nodes. The actual number of hidden layers and hidden nodes depends on the problem and the satisfactory level of the error. Hidden layers map the nonlinear relationship in the system, and the BPA permits to learning input-output representing from the training samples. The training procedure is accomplished in two passes (forward and backward) through the network. In the forward pass, input parameters are supplied to the network, and the outputs of all neurons in each layer are calculated by selecting the initial weights (arbitrarily produced values in the middle of -0.5 to +0.5). The error in the output layer is found by relating the output with the experimental value (bulk density). In the backward pass, the weights are suitably updated, so to minimize the overall training error. In doing so, two factors, called learning rate and momentum factor, are introduced for adjusting the magnitude of the weights along the descent direction. These factor values (in between 0.1 to 0.9) are chosen by trial and error method.
The ideal factors essential for the model are determined based on mean squared error (MSE) and the average error in output prediction of the test data [13]. The trend of average error of testing data sets in output prediction will help to avoid overfitting. Mean squared error of 0.001851 and average errors of 0.193 and 0.03247 (for training and testing data) was achieved after 3000 iterations with two hidden layers consist of 2 hidden nodes in each layer with the learning rate of 0.6 and momentum term of 0.4. The model with this architecture is used for prediction and analysis of the bulk density of barium titanates. The model can be used to calculate the density by performing sensitivity analysis at various process parameters within the predictive range specified in Table 1. Overview of sensitivity analysis was well documented and described in earlier publications [13-15]. The sensitivity analysis was calculated by varying one input parameter at smaller intervals within the range while keeping other elements constant and studying their influences on output.
3. Results and discussion
The performance of the model was evaluated by predicting bulk density for 20 new unseen experimental data. The comparison between the predicted and experimental density was plotted in Figure 2. As shown in Fig. 2, the correlation coefficients Pearson’s r = 0.972 and Adj. R-square = 0.945 values obtained for training data sets as the experimental and predicted values are very close to each other. Therefore, it can be understood that the trained model can be used to learn the correlation among the input and output values with the smallest error. These values indicate that the ANN model has been well trained, as shown in Fig. 2(a). As seen in Fig. 2(b), the goodness of fit between the experimental value and the predicted value from the developed model for 20 unseen testing data sets of the density of barium nitrate is found to be 0.9745 and 0.9468. Predicted values are closer to measured values for unseen 20 data sets indicating the testing ability of the ANN model. These results show the density of barium nitrate can be predicted with excellent precision by using the ANN model.
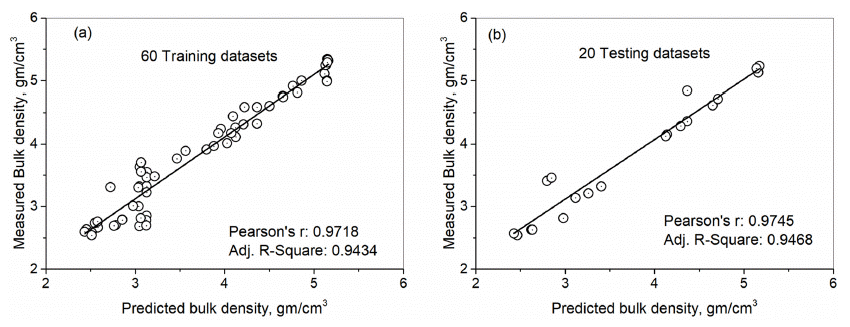
Comparison of predicted and measured the bulk density of barium titanates (a) for training data sets and (b) for testing data sets.
3.1 Transformation of Artificial Neural Networks Weights
We obtained the optimum model by varying hidden layers, (one to three), neurons in the hidden layer (one to ten), learning rate, momentum term, and the number of iterations. The optimum model was found with an architecture of 5-2-2-1, with a learning rate of 0.6 and a momentum term of 0.4 at 3000 iterations. 5-2-2-1 architecture will have (5+1)X2 + (2+1)X2 + (2+1)X1 = 12 +6 +3 = 21 network weights. Initially, these 21 weights were randomly generated between -0.5 to +0.5. After training, the weights of the ideal model were transformed to -7.83 to 3.96, as shown in Fig. 3. These transformed weights are capable of mapping the relationship between processing parameters and bulk density of barium nitrates. By using these weights, we developed a graphical user interface design for predicting the bulk density of barium nitrate, as shown in Fig. 4. We created a virtual experimental system with the mean values of the database and examined the model to predict the bulk density. The prediction is in good agreement with the existing knowledge of powder metallurgy. Here, within the ranges of five process parameters, there are infinite combinations of experiments were possible. By using this ANN model, we can predict the bulk density with a mouse click without any experimental measurement and saves a lot of resources.
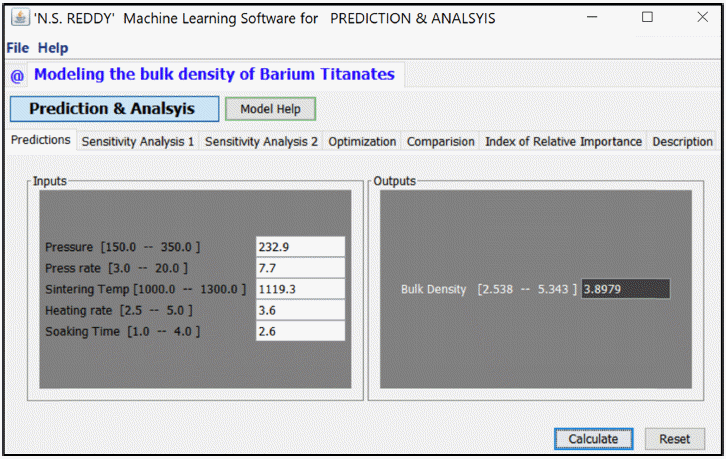
The user interface of the model based on the weights obtained from ideally trained architecture (5-2-2-1) and predictions at the mean values of the database.
The usefulness of the model is not limited only for the prediction of bulk density at new experimental conditions. It can also be used to study the impact of processing variables on the density by performing sensitivity analysis on the ANN model. Figure 5 illustrates the effect of process parameters by varying from minimum to maximum value (by using the sensitivity tab in Figure 4) and its impact on the bulk density of barium titanates by keeping other elements unchanged.

Predicted impact of process parameters on bulk density of barium titanates. Pressure: 150MPa, Rate of the press: 15 KN/S, sintering temperature: 1200°C, heating rate: 3.5°C/min, and soaking time: 1 h.
We selected a sample with process parameters of pressure: 150 MPa, rate of the press: 15 KN/S, sintering temperature: 1200°C, heating rate: 3.5°C/min, and soaking time: 1h and respective density is 3.891 gm/cm3. We systematically varied each parameter from minimum to maximum and predicted its impact on the density of barium titanates. Increasing the pressure from 150 to 350 MPa resulted in an increase in the bulk density as expected shown in Fig 5(a). We observed the relationship is linear or constant at all different process parameters. This is because, during the pressing process, the contact area between the powder particles is considerably increased. The succeeding rise in the pressure will increase the plastic deformation of powder particles and thus reducing the pore size, hence leading to a higher density. Change in the rate of the press has not influenced the density for these particular conditions. Therefore it was not shown here. Fig. 5(b) shows the variation of sintering temperature from 1000 to 1300°C and its simulated impact on density. As sintering temperature increases, the convergence between the grains leading to a reduction in pore size and making the denser barium titanates.
Figure 5(c) shows the effect of heating rate on density, as the heating rate increases the density decreases in almost all the cases irrespective of other parameters. These predictions can be attributed that at lower values of heating rate, the bath material will be evaporated, and leads to an increase in the sintering time. As a result, there will be a decrease in porosity and the subsequent increase in density and vice versa. Fig. 5(d) shows that the change in soaking time from 1 to 4 h resulted in an increase in density up to 2 h and later the density decreased marginally. In practice, the increase in the soaking time will make the grains converge more, and this will reduce the porosity and increase the density. The predicted effect of soaking time also depends on the values of the other process parameters. In some cases, the predictions are in good agreement with the earlier works and existing knowledge. However, the forecasts with this particular combination of process parameters resulted in a marginal decrease in density after two hours of soaking time, which are contradictory with earlier work and need further study.
4. Conclusions
The predictions with unseen experimental data show (high correlation coefficients) that the neural networks can model complicated and non-linear complex relationships between bulk density and process parameters of barium titanates. The sensitivity analysis helps to identify the effect of process parameters and the predictions are in good agreement with powder metallurgy principles. The predictions by the model provide useful information from relatively small experimental databases (60 data sets). On the shop-floor, a great deal of time, energy and materials can be saved to find the bulk density of an unknown combination of process parameters within the range. The developed model can save experimental resources from estimating the density of barium titanates.
Acknowledgments
NSR acknowledges YSJ – GKK for the inspiration and YKK for the model development.